Weekly Recap (Sep 2024, part 1)
What I'm reading this week in de-extinction and patent law, ATAC-seq benchmarking, large animal genomes, SV genotyping with long reads, cell type-specific enhancer prediction, AI in biosecurity, ...
This week’s recap highlights perspectives in de-extinction and patent law, systematic benchmarking of scATAC-seq methods, a 91 gigabase (!) animal genome, structural variant genotyping with long reads, cell type-specific enhancer prediction, and a perspective piece in AI in biosecurity.
Others that caught my attention include a new lossless compression tool for VCF files, benchmarking DNA foundation models for genomic sequence classification, an interactive pangenome knowledge base, Dodo systematics, a perspective on near/mid-term risks and opportunities of open-source generative AI, gene ontology assignment using protein similarity, a chatbot for talking to protein sequences and getting answers about their functions, BGI’s new single nanopore sequencer, and a real-time plasmid transmission detection pipeline.
Deep dive
The patentability and bioethics of molecular de-extinction
This one caught my attention as it intersects two areas I’m interested in: patent law and de-extinction. Back in the 00s I took the patent bar exam (you can do this without going to law school), thinking that I might want to go into patent law in genomics after grad school. I watched the SCOTUS docket closely to see how the court would rule in the AMP vs Myriad case, and followed the now-defunct Genomics Law Report for actual expert analysis on arguments and evidence. Myriad had patented the sequence of the BRCA1 gene, and methods for assessing cancer risk with genetic testing. The Wikipedia article on the Myriad case is a great read, but the short story of the ruling was this: the court ruled that “a naturally occurring DNA segment is a product of nature and not patent eligible merely because it has been isolated,” but made a concession that a cDNA sequence identical to the protein-coding sequence of the same gene can be patented, reasoning that a cDNA sequence is synthetic and isn’t naturally occurring in nature. This article explores the patentability of sequences derived from de-extinction research. If I stumble across well-preserved DNA from a woolly indricothere (a giraffe-sized hornless hairy rhinoceros), and I sequence then synthesize genes involved in body size — are those sequences patentable? They aren’t naturally occurring, but they were — during the middle Eocene to late Oligocene epochs, tens of millions of years ago. Current patent law couldn’t have imagined such a scenario, and the answer will likely need to be legislative, not judicial.
Paper: Torrance, Andrew W., and Cesar de la Fuente-Nunez. “The patentability and bioethics of molecular de-extinction.” Nature Biotechnology, 2024. DOI: 10.1038/s41587-024-02332-x. Read free: https://rdcu.be/dRyXd.
TL;DR: This article explores the patentability and bioethical implications of molecular de-extinction, a novel approach enabled by AI and machine learning to resurrect extinct molecules for modern applications, raising questions about the intersection of patent law and ethical considerations.
Summary: The article examines the emerging field of molecular de-extinction, which uses sequencing, bioinformatics, and synthetic biology to resurrect extinct molecules, offering a new avenue for addressing contemporary challenges without reviving entire organisms. The authors discuss the patentability of such molecules, comparing them to naturally occurring DNA and synthetic molecules in light of the 2013 Myriad decision by the US Supreme Court, which ruled that naturally occurring DNA cannot be patented. The article suggests that extinct molecules may be patentable due to their non-natural occurrence and the human ingenuity required to infer and synthesize them. The authors emphasize the need for careful consideration of these issues as the field of molecular de-extinction advances, balancing innovation with ethical responsibilities.
Highlights:
Patent Law Analysis: Examines the potential patentability of extinct molecules through the lens of the Myriad decision and patent law doctrine.
Comparison with Synthetic Molecules: Explores parallels between extinct and synthetic molecules in terms of patent eligibility.
Bioethical Framework: Discusses the broader ethical implications of resurrecting extinct molecules, particularly in relation to ecosystem preservation and scientific progress.
Legal and Ethical Guidelines: Proposes considerations for policymakers and patent offices to balance innovation with ethical concerns in the context of molecular de-extinction.
Case Studies: References multiple studies and legal precedents to support the analysis, offering a comprehensive overview of the current landscape.
Benchmarking computational methods for single-cell chromatin data analysis
The number of tools/software/methods for single-cell analysis is bewildering. It’s great to see thoughtful and systematic benchmarking analyses performed by independent groups with no dog in the fight. In the last post I covered the recent spatial transcriptomics benchmarking paper. This one covers methods for ATAC-seq.
Paper: Luo, Siyuan, et al. “Benchmarking computational methods for single-cell chromatin data analysis.” Genome Biology, 2024. DOI:10.1186/s13059-024-03356-x.
TL;DR: This study benchmarks eight feature engineering pipelines derived from five methods for analyzing single-cell chromatin accessibility data, identifying SnapATAC2 as the best method for complex datasets and SnapATAC2 and aggregation as the most scalable methods for large datasets.
Summary: The paper provides a comprehensive benchmark of computational methods for analyzing single-cell chromatin accessibility data, focusing on scATAC-seq and related multi-omics datasets. It evaluates eight feature engineering pipelines derived from five popular methods: Signac, ArchR, SnapATAC, SnapATAC2, and an aggregation method. The study uses six diverse datasets with varying complexities and sequencing protocols to assess the performance of each method at different data processing stages, including feature engineering, dimensional reduction, and clustering. SnapATAC2 and aggregation methods consistently outperform others in identifying cell types, especially in complex datasets with hierarchical structures. SnapATAC2 also excels in scalability, making it suitable for large datasets. The findings provide guidelines for choosing appropriate methods based on dataset characteristics and highlight areas for future method development, such as mitigating library size biases and improving the handling of rare cell types.
Highlights:
SnapATAC2 Superiority: Demonstrated the best performance in complex datasets with hierarchical clustering structures.
Aggregation Strategy: Showed top performance in simple datasets with distinct cell types and is second best in more complex datasets.
Scalability: SnapATAC2 and aggregation methods are the most scalable, handling large datasets efficiently with minimal memory and time consumption.
Benchmarking Pipeline: The benchmarking workflow is available as a Snakemake pipeline: https://github.com/RoseYuan/sc_chromatin_benchmark. Notebooks, R scripts, and supporting data used for preprocessing datasets and generating all the visualizations in this manuscript is available at https://github.com/RoseYuan/benchmark_paper.
Data availability: For the analyzed datasets, the preprocessed data that can be directly input into the Snakemake pipeline is available on Zenodo at https://doi.org/10.5281/zenodo.8212920. For the unprocessed data, fragment files of the cell line dataset were downloaded from GEO accession GSE162690, fragment file and the gene expression matrix file of the 10X PBMC multiomics dataset were downloaded from 10X Genomics, and fragment files of the human adult atlas datasets were downloaded from GEO accession GSE184462. Additional datasets used in the study are described in the Availability of data and materials section.
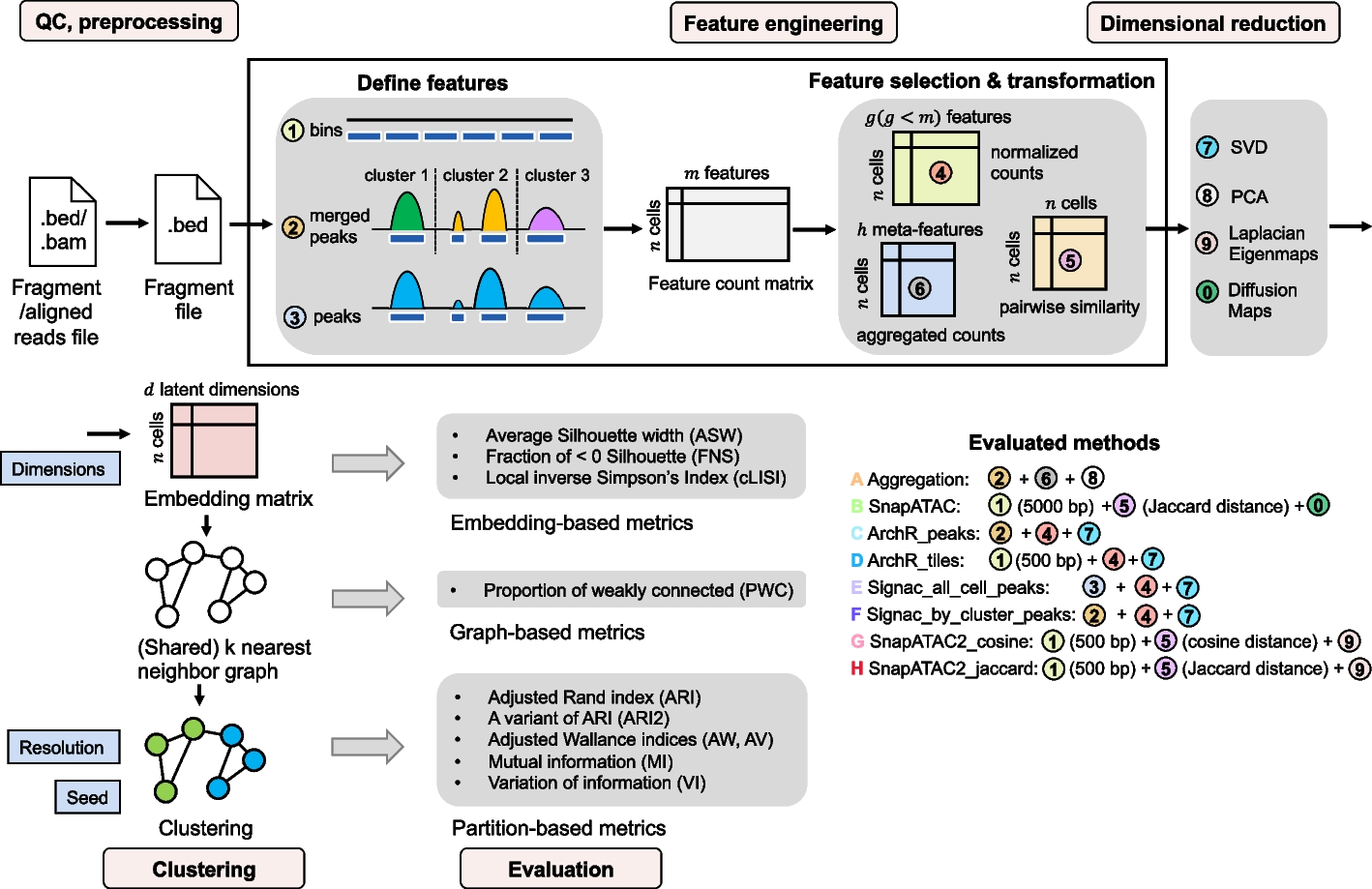
The genomes of all lungfish inform on genome expansion and tetrapod evolution
This represents the largest animal genome ever sequenced at 91 Gb — about 30 times the size of the human genome. 18 of 19 chromosomes are each individually larger than the entire 3 Gb human genome.
Paper: Schartl, Manfred, et al. “The genomes of all lungfish inform on genome expansion and tetrapod evolution.” Nature, 2024. DOI:10.1038/s41586-024-07830-1. (Free to read: https://rdcu.be/dRE64).
TL;DR: This study explores the genomes of all extant lungfish species, revealing insights into genome expansion, transposable element activity, and the evolutionary processes leading to the water-to-land transition in vertebrates, offering a detailed look at the molecular basis of tetrapod evolution.
Summary: The research presents a comprehensive analysis of the genomes of the three living lungfish species: African, South American, and Australian. The South American lungfish has the largest animal genome sequenced to date, at approximately 91 Gb, mainly due to transposable elements (TEs). The study highlights how TE activity continues to drive genome expansion in lungfish, especially in the South American species. Despite their massive and expanding genomes, lungfish chromosomes remain highly conserved, reflecting the ancestral tetrapod karyotype. The study also examines the loss of limb-like appendages in African and South American lungfishes, attributing it to the loss of specific sonic hedgehog limb enhancers and other gene regulatory elements. These findings provide crucial insights into the genomic and molecular mechanisms underlying the evolutionary transition from water to land in vertebrates, particularly the conservation of synteny and the role of genome size in evolutionary adaptation.
Methodological highlights:
Chromosome-Level Assembly: Achieved through long-read sequencing and Hi-C scaffolding, resulting in highly complete assemblies of all lungfish genomes.
Transposable Element Analysis: Identified active TEs driving genome expansion, particularly LINEs, and the role of piRNA pathway deficiencies in this process.
Comparative Genomics: Explored synteny conservation across lungfish genomes, providing evidence for the retention of ancestral tetrapod chromosomal structures.
New tools, data, and resources:
Genome Assemblies: Genome assemblies and sequencing data are available from NCBI Bioprojects PRJNA808321, PRJNA808322, PRJNA813994, PRJNA813995 and PRJNA981572 and at BioSamples SAMN26083907 and SAMN26533844. Gene and repeat annotations are available at Figshare.
Code: Code for the analysis is spread across several repositories:
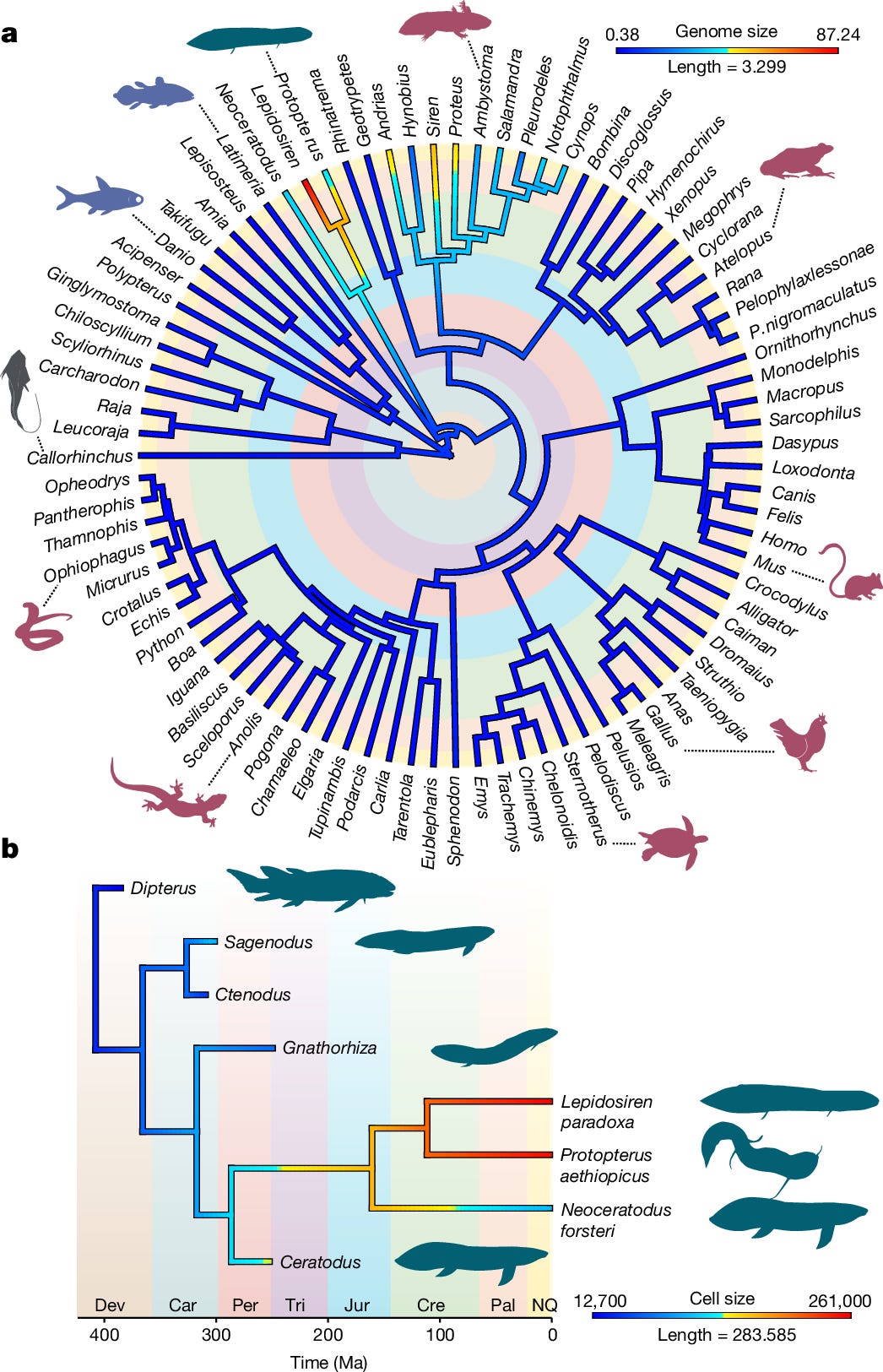
Sawfish: Improving long-read structural variant discovery and genotyping with local haplotype modeling
The lead author, Chris Saunders, was previously at Illumina and developed Strelka2 (paper, code). I and my team used Strelka2 extensively in a previous job when GATK wasn’t licensed for commercial use. This one caught my attention with Chris Saunders as the lead author along with a list of other star bioinformaticians at PacBio.
Paper: Saunders, Christopher T., et al. “Sawfish: Improving long-read structural variant discovery and genotyping with local haplotype modeling.” bioRxiv, 2024. DOI:10.1101/2024.08.19.608674.
TL;DR: Sawfish is a new SV caller that enhances long-read structural variant (SV) discovery by incorporating local haplotype modeling, achieving superior accuracy and resolution compared to other state-of-the-art tools across various SV sizes and sequencing depths.
Summary: Sawfish introduces a new method for detecting structural variants (SVs) by utilizing local haplotype modeling in long-read sequencing data. This approach systematically models SV haplotypes during both discovery and genotyping, which significantly improves the accuracy of SV calls, particularly in complex regions of the genome. Sawfish was benchmarked against the Genome in a Bottle (GIAB) T2T-HG002-Q100 diploid assembly and other SV callers like Sniffles2 and pbsv, demonstrating the highest accuracy across all tested SV size groups and sequencing depths. It also showed superior performance in detecting SVs in medically relevant genes, achieving the highest genotype concordance in a large pedigree study. These results suggest that Sawfish sets a new standard for SV discovery and genotyping, particularly in high-coverage, long-read datasets.
Methodological highlights:
Local Haplotype Modeling: Integrates haplotype information during SV discovery and genotyping, improving call accuracy, especially in complex regions.
Benchmarking with GIAB T2T: Sawfish demonstrated the highest F1-score across all tested SV sizes, outperforming other leading SV callers like Sniffles2 and pbsv.
Joint Genotyping: Provides more accurate joint-genotyping results, with higher concordance rates in pedigree analyses compared to other methods.
New tools, data, and resources:
Sawfish Software: Available at https://github.com/PacificBiosciences/sawfish. The tool is a pre-compiled Linux binary, optimized for high-accuracy, long-read sequencing data. Note that the GitHub page doesn’t have the actual source code — only binaries are available on the release page.
Data availability: The paper used publicly available HiFi WGS data for HG002, sequenced to ~32-fold coverage on the Revio system. The unmapped sequencing data can be downloaded from https://downloads.pacbcloud.com/public/revio/2022Q4/HG002-rep1/m84011_220902_175841_s1.hifi_reads.bam.
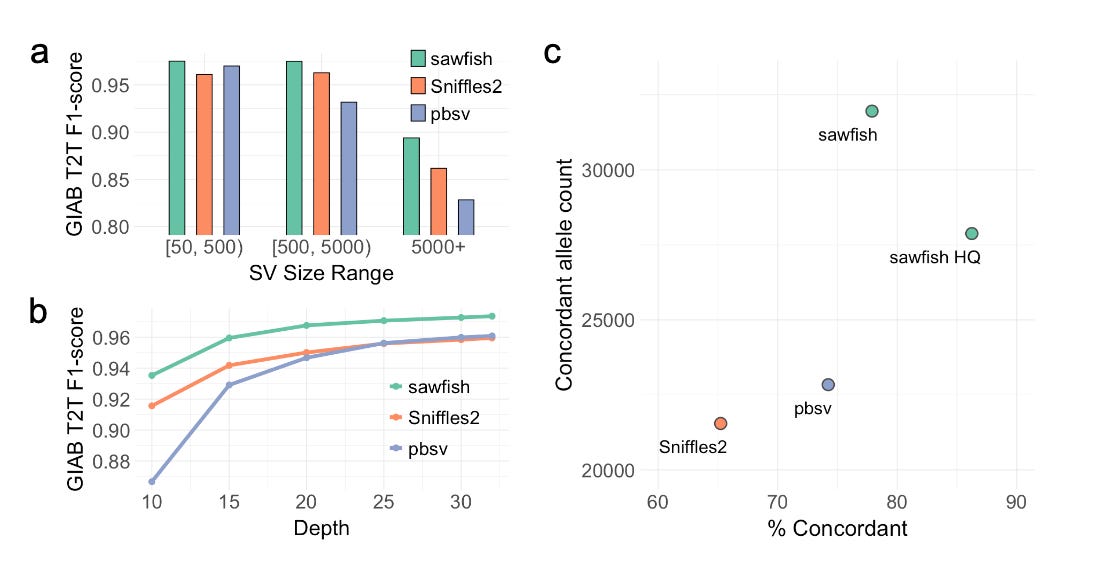
Evaluating Methods for the Prediction of Cell Type-Specific Enhancers in the Mammalian Cortex
Paper: Johansen, et al. “Evaluating Methods for the Prediction of Cell Type-Specific Enhancers in the Mammalian Cortex.” bioRxiv, 2024. DOI: 10.1101/2024.08.21.609075.
TL;DR: This study presents a community-driven challenge to evaluate computational methods for predicting cell type-specific enhancers in the mammalian cortex, revealing that ATAC-seq specificity is a key predictor of functional enhancers and that combining sequence models with ATAC-seq data improves prediction accuracy.
Summary: The paper discusses the results of the BICCN Challenge, which assessed various computational methods for predicting cell type-specific enhancers in the mouse cortex using cross-species multi-omics data. The challenge involved six teams employing different approaches, including machine learning and feature-based methods, to predict the functionality of enhancers. The study found that methods leveraging ATAC-seq specificity performed best, particularly when combined with sequence models that could identify transcription factor motifs. The top-performing teams demonstrated that while chromatin accessibility is a strong predictor of enhancer activity, additional features like histone modifications and transcription factor motifs further refine predictions. The challenge sets a benchmark for enhancer prediction, contributing to the development of more accurate tools for genetic research in neuroscience. The methods section includes a description of each team’s approach, and is a good read for anyone interested in methods for cell type-specific enhancer prediction.
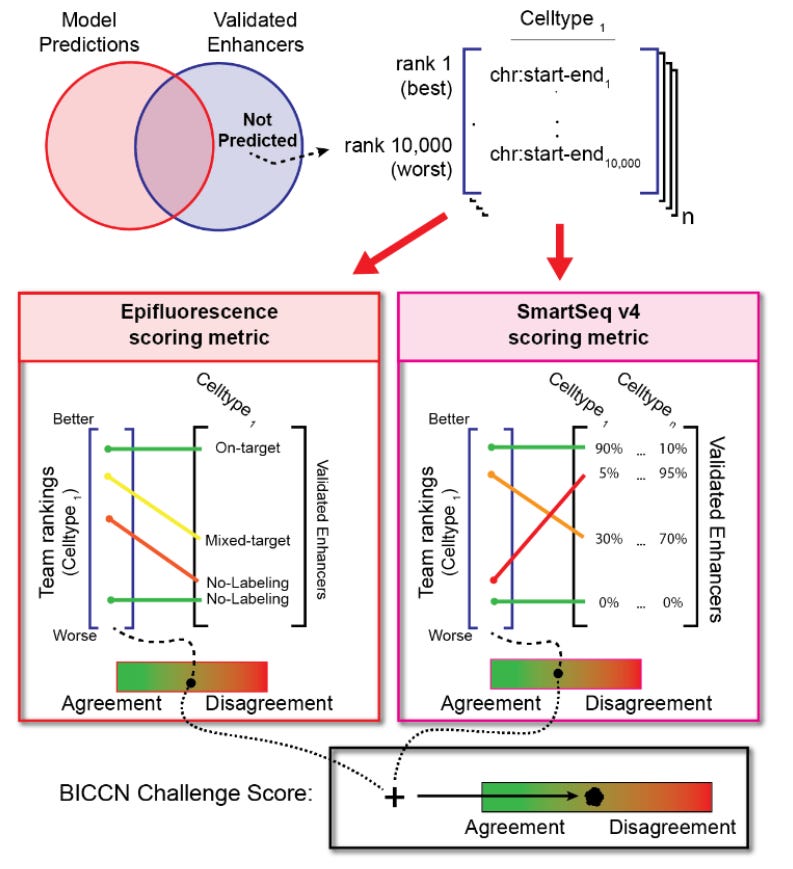
AI and biosecurity: The need for governance
Prior to coming to Colossal+Form last year, I worked in biosecurity for over a decade in academia and industry/government. AI models trained on biological data are dual use and could potentially be used to create or enhance bioweapons. A lot of ink has been spilled on this topic. The RAND corporation published a report back in January — The Operational Risks of AI in Large-Scale Biological Attacks: Results of a Red-Team Study — where they concluded that planning a biological weapon attack lies beyond the capability of current frontier LLMs, finding little significant difference between the viability of attack plans generated with or without LLMs. In this Policy Forum perspective piece, the authors propose government regulations requiring models trained on biological data to be evaluated before release (although it’s unclear what “evaluation” means or who would perform the evaluation). Additionally, the authors call for further legislation regulating DNA synthesis screening (there’s already a requirement that federally funded researchers order their DNA from synthesis providers who self-attest that they implement a screening program).
Paper: Bloomfield, Doni, et al. “AI and biosecurity: The need for governance.” Science, 2024. DOI: 10.1126/science.adq1977.
TL;DR: The article discusses the intersection of artificial intelligence (AI) and biosecurity, emphasizing the dual-use risks posed by advanced AI models that can manipulate biological data. While AI holds promise for breakthroughs in medicine and agriculture, it also presents significant biosecurity risks, such as the potential creation of dangerous pathogens. The authors advocate for a regulatory framework to govern the development and deployment of these models, proposing mandatory oversight by governments to prevent misuse. They also highlight the need for international cooperation and the establishment of standardized safety evaluations, balancing scientific innovation with the imperative to prevent catastrophic biological threats.
Other papers of note
GSC: efficient lossless compression of VCF files with fast query https://academic.oup.com/gigascience/article/doi/10.1093/gigascience/giae046/7716932 https://github.com/luo-xiaolong/GSC
Benchmarking DNA Foundation Models for Genomic Sequence Classification https://www.biorxiv.org/content/10.1101/2024.08.16.608288v1
PanKB: An interactive microbial pangenome knowledgebase for research, biotechnological innovation, and knowledge mining https://www.biorxiv.org/content/10.1101/2024.08.16.608241v1?rss=1 https://github.com/biosustain/pankb
Systematics and nomenclature of the Dodo and the Solitaire https://academic.oup.com/zoolinnean/article/201/4/zlae086/7733394
Near to Mid-term Risks and Opportunities of Open-Source Generative AI https://arxiv.org/abs/2404.17047
DIAMOND2GO: A rapid Gene Ontology assignment and enrichment tool for functional genomics https://www.biorxiv.org/content/10.1101/2024.08.19.608700v1
ProteinChat: Multi-Modal Large Language Model Enables Protein Function Prediction https://www.biorxiv.org/content/10.1101/2024.08.19.608729v1
CycloneSEQ: BGI's new single-molecule nanopore sequencing platform https://www.biorxiv.org/content/10.1101/2024.08.19.608720v1?rss=1
Real-time Plasmid Transmission Detection Pipeline https://www.biorxiv.org/content/10.1101/2024.07.09.602722v2