What I'm reading (Aug 2024, part 3)
What I'm reading this week in structural variant analysis, population/medical genetics, infectious disease modeling+forecasting, ML in single cell omics, paleogenetics, pangenomes, cancer genomics ...
Here are a few papers that caught my attention recently. I summarize a few in the deep dive at the top, then link to a few other papers of note later on. Subscribe to Paired Ends to get periodic summaries like this delivered to your e-mail.
Deep dive
Evaluation of FluSight influenza forecasting in the 2021-22 and 2022-23 seasons with a new target laboratory-confirmed influenza hospitalizations
A bit of shameless self-promotion here: I and several of my colleagues at my last company were co-authors on this large consortium effort that was just published. I was co-PI on a grant from CSTE (subaward from CDC) to develop methods for forecasting flu hospitalizations. We had previously participated in related consortia efforts for forecasting COVID-19 and influenza cases, but lab-confirmed flu hospitalizations were a new target for these seasons. Forecasting influenza-like illness (ILI) got really complicated after COVID-19 disrupted the typical seasonality of ILI burden (I wrote this paper about that problem). This paper describes the consortium effort, the ensemble model across all forecasters, and describes how the model performs over different periods of the flu season.
Paper: Mathis, et al. “Evaluation of FluSight influenza forecasting in the 2021–22 and 2022–23 seasons with a new target laboratory-confirmed influenza hospitalizations.” Nature Communications, 2024. DOI:10.1038/s41467-024-50601-9.
TL;DR: This study evaluates the performance of FluSight ensemble and individual models in forecasting laboratory-confirmed influenza hospitalizations during the 2021–22 and 2022–23 seasons, demonstrating the effectiveness of the ensemble model but highlighting challenges during periods of rapid change.
Summary: The paper assesses the accuracy and reliability of the FluSight ensemble and its component models in predicting weekly laboratory-confirmed influenza hospital admissions across the United States for the 2021–22 and 2022–23 seasons. The study reveals that while the FluSight ensemble performed robustly overall, especially compared to individual models, its performance declined during periods of rapid change, such as sharp increases in hospitalizations. The analysis used the Weighted Interval Score (WIS) to measure forecast skill and found that the ensemble model generally outperformed the baseline but faced challenges over longer forecast horizons. The paper underscores the importance of continuing to refine forecasting models, particularly to better anticipate changes during atypical influenza seasons.
Methodological highlights:
Weighted Interval Score (WIS): Utilized to evaluate forecast skill, comparing the ensemble model’s performance to both the baseline and individual models.
Forecast Horizon Analysis: Assessed model performance over 1- to 4-week horizons, revealing a decline in accuracy over longer periods.
Spatial Analysis: Examined forecast accuracy across different U.S. states and territories, noting variability in performance.
FluSight Forecasts: Data and models from the study are publicly available and can be accessed through the CDC’s FluSight website: https://www.cdc.gov/flu/weekly/flusight/index.html.
Interactive Dashboard: The study includes an interactive dashboard for visualizing forecast data, accessible at https://zoltardata.com/project/299/viz.
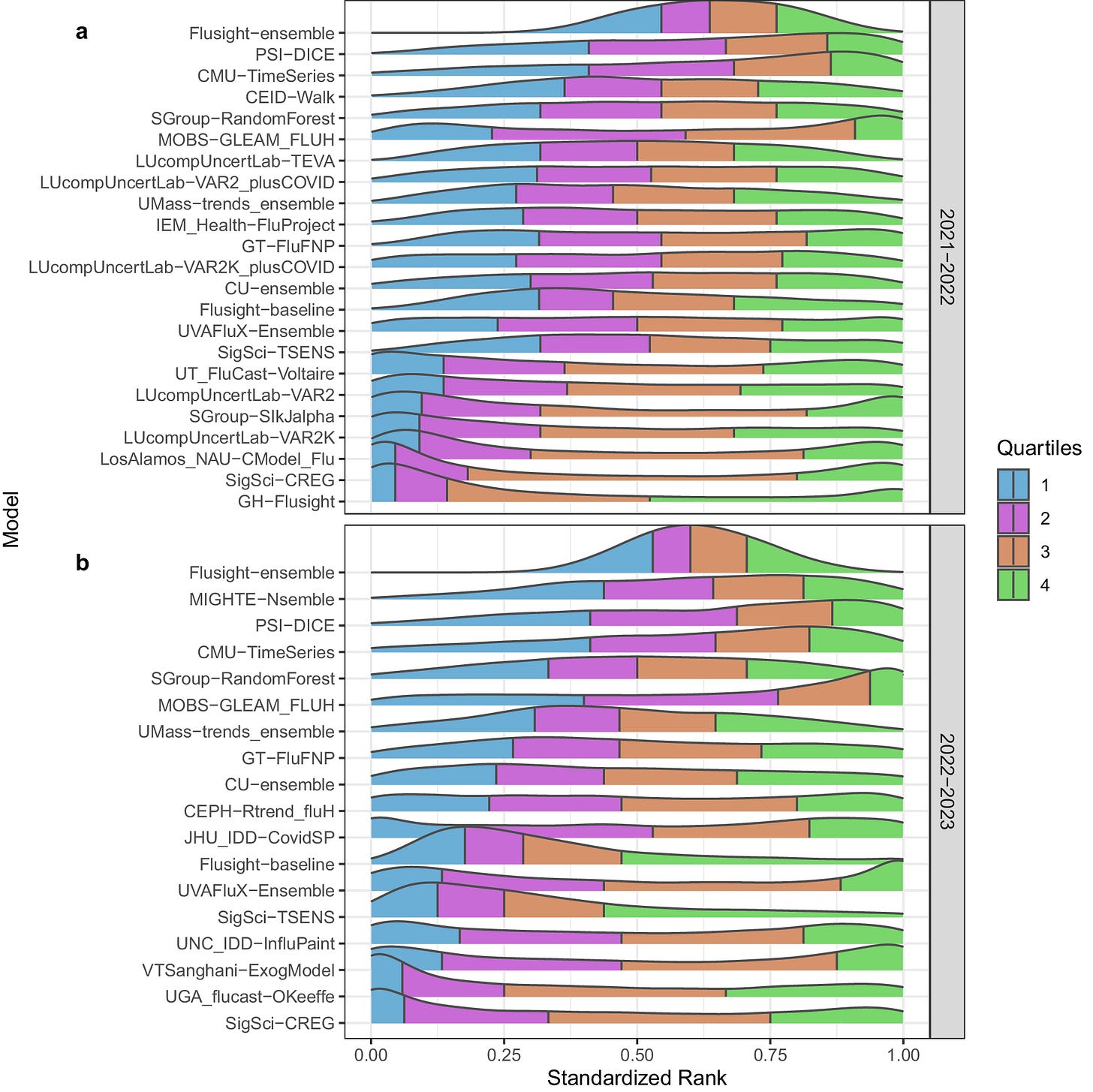
A Comparison of Structural Variant Calling from Short-Read and Nanopore-Based Whole-Genome Sequencing Using Optical Genome Mapping as a Benchmark
Paper: Pei, Yang, et al. “A Comparison of Structural Variant Calling from Short-Read and Nanopore-Based Whole-Genome Sequencing Using Optical Genome Mapping as a Benchmark.” Genes, 2024, 15, 925. DOI:10.3390/genes15070925.
TL;DR: This study evaluates the effectiveness of structural variant (SV) calling using Illumina short read sequencing, ONT long read sequencing, and Bionano optical genome mapping (OGM). It shows that OGM has high precision and that Nanopore with Sniffles2 improves sensitivity significantly over short-read sequencing.
Summary: The paper compares the performance of three genomic technologies — Illumina short-read sequencing, Oxford Nanopore long-read sequencing, and Bionano optical genome mapping (OGM) — in detecting structural variants (SVs). Using a cohort of 9 parent-child trios, the study benchmarks SV calls against a “truth” dataset established from OGM. The results indicate that OGM has a high precision (95%) in detecting SVs. Illumina sequencing showed high sensitivity for deletions but poor for insertions. The performance of Nanopore sequencing improved substantially with the Sniffles2 variant caller, demonstrating superior sensitivity over Illumina for most SV types, particularly insertions. The study highlights the potential of OGM and improved Nanopore sequencing methods in clinical genomics for detecting rare SVs.
Methodological highlights:
High Precision of OGM: Demonstrated 95% precision in detecting rare SVs.
Variant Calling Sensitivity: Illumina showed 86% sensitivity for deletions but only 22% for insertions. Nanopore with Sniffles2 showed 90% sensitivity for deletions and 74% for insertions.
Benchmarking with a Truth Dataset: Used OGM calls as a reference to assess the performance of SV callers in other technologies.
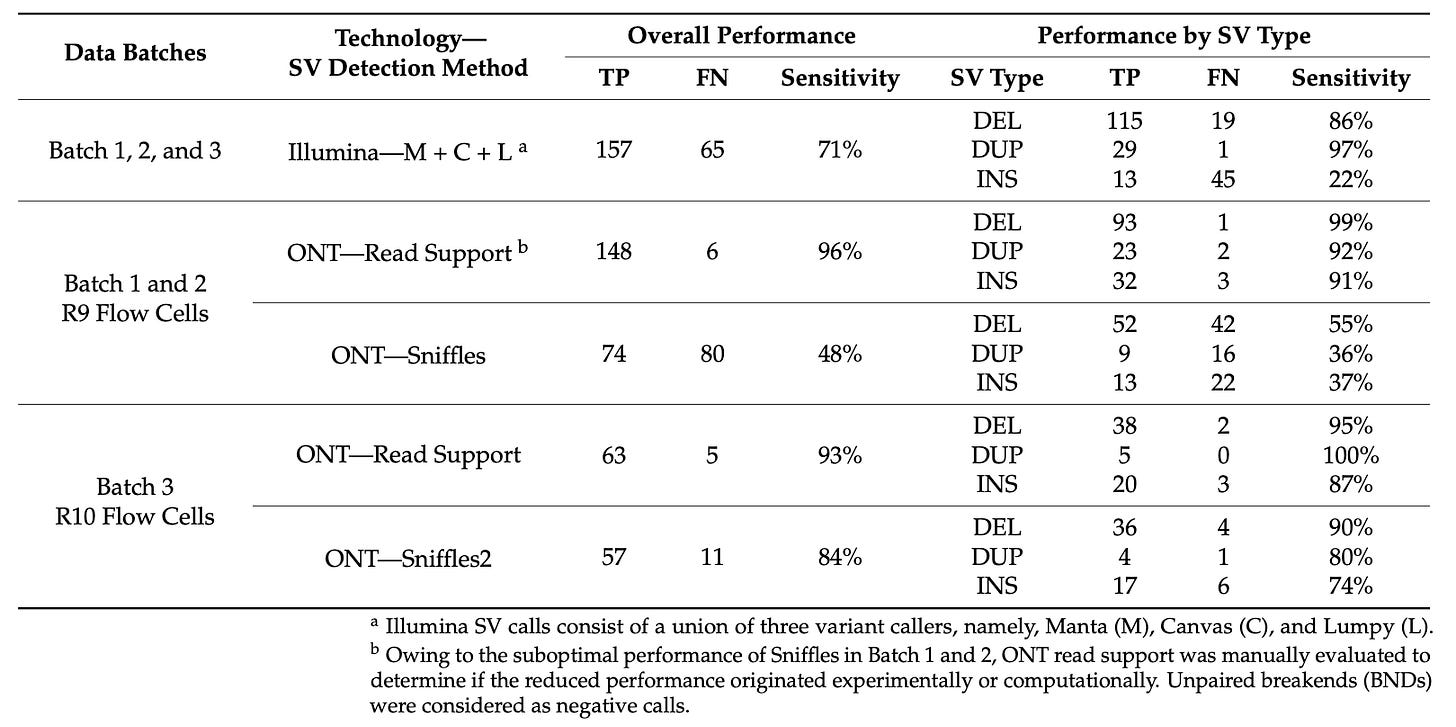
Promoting equity in polygenic risk assessment through global collaboration
Paper: Kullo, Iftikhar J. “Promoting equity in polygenic risk assessment through global collaboration.” Nature Genetics, 2024. DOI:10.1038/s41588-024-01843-2. ReadCube (read free): https://rdcu.be/dP1lC.
TL;DR: This perspective paper emphasizes the need for global collaboration to develop equitable polygenic risk scores (PRSs) that perform well across diverse populations, addressing current disparities due to the limited representation of non-European ancestry groups in genomic studies. I included this paper in this week’s deep dive because the reference list is so useful and recent.
Summary: The perspective article discusses the challenges and opportunities in developing equitable polygenic risk scores (PRSs) to improve disease prediction and prevention globally. PRSs, which aggregate genetic variants to estimate disease risk, often perform poorly in non-European populations due to the Eurocentric focus of genome-wide association studies (GWAS). The author calls for global collaboration to generate new data in low- and middle-income countries (LMICs), enhance diversity in GWAS datasets, and develop new statistical methods for PRS construction. Key actions include building biobanks, sharing data securely, and ensuring ethical, legal, and social considerations are met. The article underscores the importance of involving local researchers and communities in genomic research and implementing PRSs in clinical settings to reduce health disparities.
Perspective highlights:
Global Data Generation: Emphasizes the need to establish new biobanks and perform genotyping/sequencing in diverse populations.
Data Sharing: The perspective highlights the importance of data sharing through initiatives like the Global Biobank Meta-analysis Initiative (GBMI) and the Polygenic Score Catalog, and advocates for the use of Trusted Research Environments (TREs) and standardized data-sharing protocols to facilitate global collaboration.
Method Development: Calls for innovative statistical methods to improve PRS performance across different ancestries, including local ancestry mapping and graph genomes.
Biobanks and Genomic Initiatives: Includes references to several global biobanks and genomic initiatives aimed at increasing diversity in genomic studies, such as H3Africa, the Uganda Genome Resource, and the Qatar Genome Project.
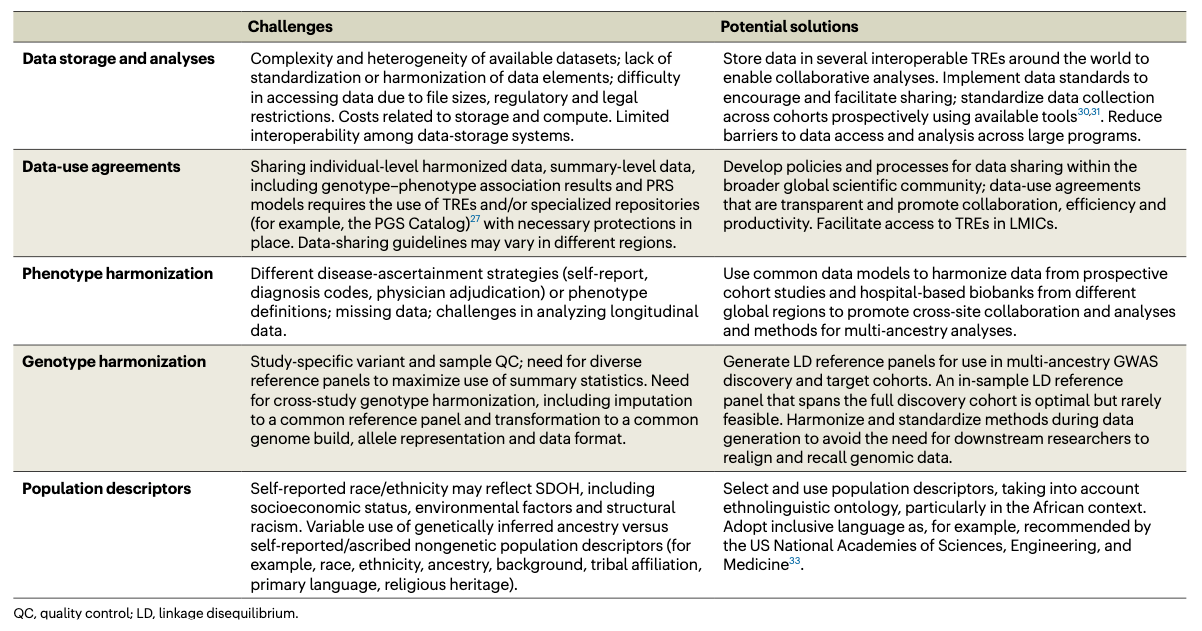
Holimap: an accurate and efficient method for solving stochastic gene network dynamics
Paper: Jia, et al. “Holimap: an accurate and efficient method for solving stochastic gene network dynamics.” Nature Communications, 2024. DOI:10.1038/s41467-024-50716-z.
TL;DR: Holimap is a novel method that accurately and efficiently predicts the stochastic dynamics of gene networks by approximating complex systems with simpler linear reaction systems, offering computational advantages over conventional methods. This method is particularly useful for studying the precise coordination and control of gene expression within intricate gene-gene interactions.
Summary: This paper introduces Holimap, a high-order linear-mapping approximation method for predicting the stochastic time-dependent dynamics of gene regulatory networks. Holimap approximates the distributions of gene product numbers in complex networks by mapping them to simpler linear systems, significantly reducing computational time while maintaining accuracy. The authors demonstrate Holimap’s effectiveness across various gene networks, including autoregulatory loops and complex randomly connected networks. Holimap outperforms conventional methods such as the stochastic simulation algorithm (SSA) and the finite-state projection (FSP) algorithm, providing noise-free and accurate predictions.
Methodological highlights:
High-Order Linear-Mapping Approximation (Holimap): Transforms complex nonlinear gene networks into simpler linear ones, enabling efficient and accurate prediction of protein number distributions.
Conditional Moment-Matching: Uses conditional moment-matching to determine effective reaction rates for the linear network, preserving key dynamic properties of the original system.
Hybrid Method: Combines Holimap with SSA to further enhance computational efficiency while maintaining high accuracy.
Holimap Software: The Holimap software is implemented in Matlab, available on GitHub (note, no license specified): https://github.com/chenjiacsrc/Holimap.
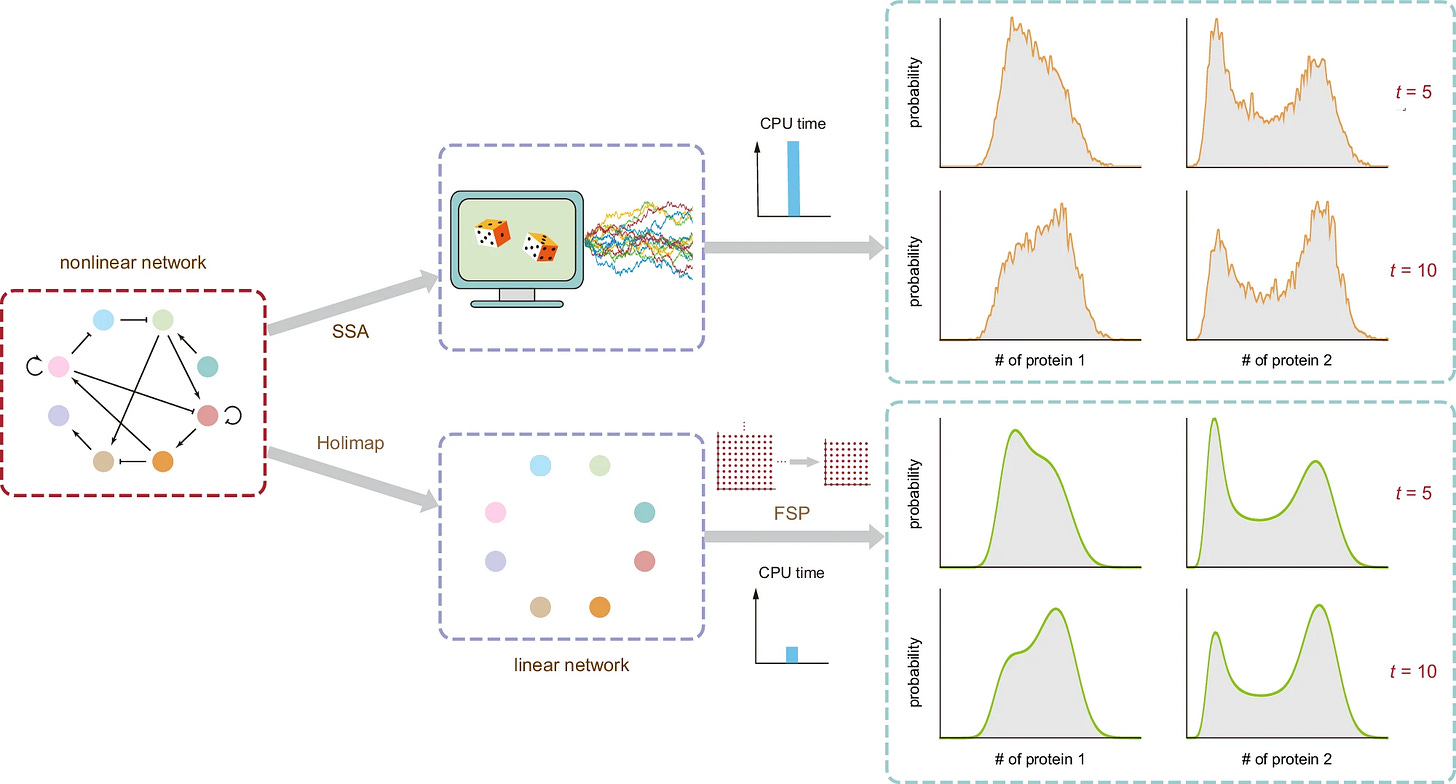
Genetic risk factors for COVID-19 and influenza are largely distinct
Paper: Kosmicki, et al. “Genetic risk factors for COVID-19 and influenza are largely distinct.” Nature Genetics, 2024. DOI:10.1038/s41588-024-01844-1.
TL;DR: This study identifies distinct genetic risk factors for COVID-19 and influenza through a large-scale genome-wide association study (GWAS), showing minimal overlap in genetic susceptibility between the two diseases.
Summary: This research explores the genetic underpinnings of susceptibility to COVID-19 and influenza, revealing largely distinct genetic risk factors for each. A genome-wide association study (GWAS) of 18,334 influenza cases and 276,295 controls identified significant associations with variants in the genes B3GALT5 and ST6GAL1. These findings were replicated in independent cohorts, confirming the genes’ roles in influenza susceptibility. The study also assessed previously identified COVID-19 risk variants, finding no significant overlap with influenza risk, except for a variant in the ABO gene with opposite effects on the two diseases. These results highlight the unique genetic architecture of susceptibility to these viral infections and suggest potential therapeutic targets, such as the modulation of sialic acid on the cell surface, which impacts viral entry.
Methodological highlights:
Genome-Wide Association Study (GWAS): Conducted on a large dataset from the AncestryDNA cohort, including over 18,000 influenza cases and 276,000 controls.
Replication and Validation: Findings were validated in seven biobanks comprising over 1.1 million individuals, ensuring robust and reproducible results.
Functional Studies: siRNA knockdown of ST6GAL1 in vitro reduced influenza infectivity, supporting its role in the disease mechanism.
GWAS Summary Statistics: Available via the GWAS Catalog (accession no. GCST90432107) for further research and validation.
Data availability: Individual-level exome sequencing, genotype, and phenotype data accessible through the UK Biobank (https://www.ukbiobank.ac.uk/enable-your-research). The FinnGen release 8 influenza GWAS summary statistics are available to approved researchers at https://www.finngen.fi/en/access_results.
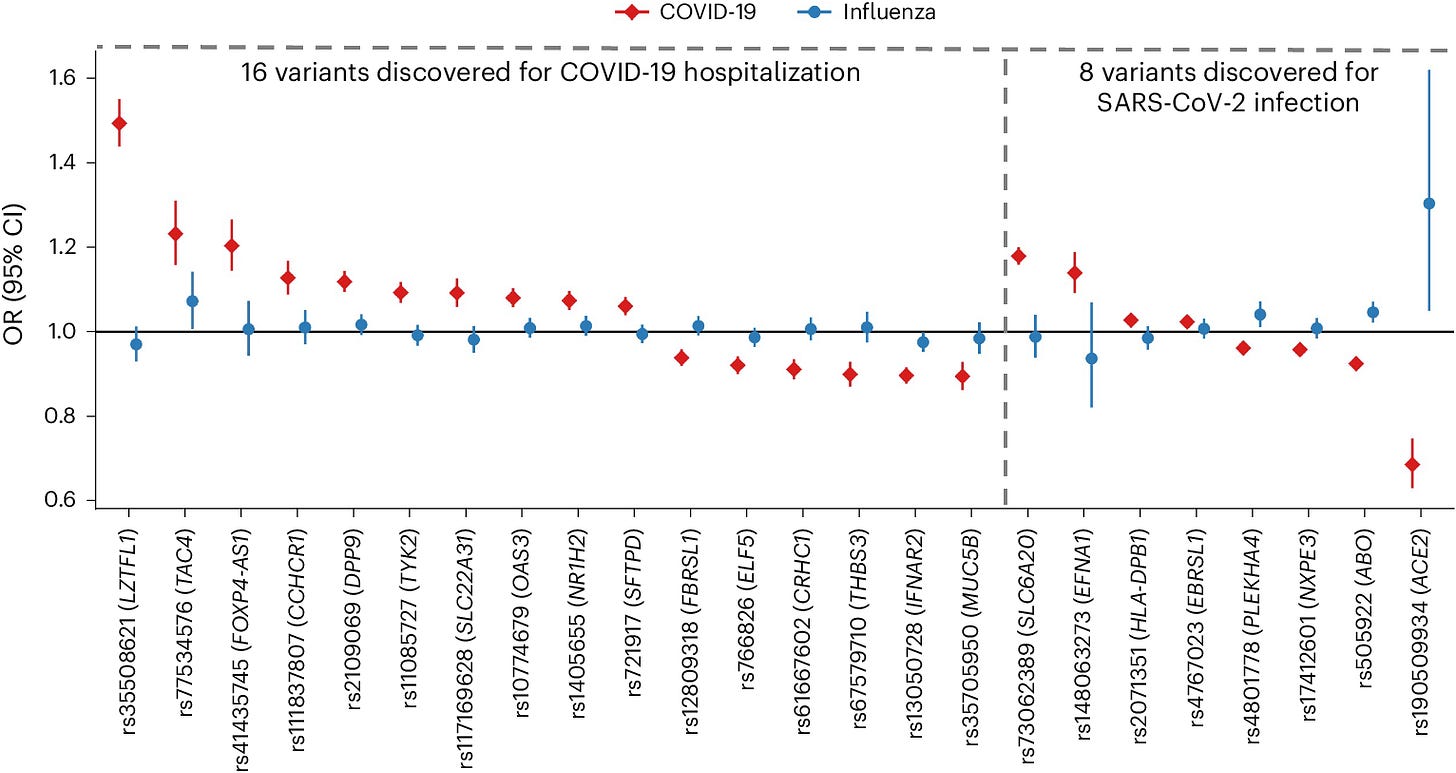
Deciphering cancer genomes with GenomeSpy: a grammar-based visualization toolkit
Paper: Lavikka, et al. “Deciphering cancer genomes with GenomeSpy: a grammar-based visualization toolkit.” GigaScience, 2024. DOI:10.1093/gigascience/giae040.
TL;DR: GenomeSpy is a versatile, GPU-accelerated toolkit for creating custom interactive visualizations of genomic data, particularly useful for cancer research. It simplifies the development process using a declarative grammar and supports the analysis of large genomic datasets.
Summary: This paper introduces GenomeSpy, a JavaScript-based toolkit designed to create tailored interactive visualizations for genomic data analysis. GenomeSpy uses a grammar-based approach, allowing users to define visualizations declaratively with JSON specifications. Its GPU-accelerated rendering ensures smooth interactions, making it ideal for exploring large datasets. The toolkit’s flexibility and performance are demonstrated through an analysis of 753 ovarian cancer samples from the DECIDER clinical trial, revealing significant insights into the genomic architecture of high-grade serous ovarian carcinoma (HGSC). GenomeSpy supports a wide range of genomic data formats and can be embedded in web applications, enhancing the accessibility and usability of genomic data visualization.
Methodological highlights:
Grammar-Based Visualization: Utilizes a JSON-based grammar inspired by Vega-Lite to define visualizations, allowing for easy customization and reuse.
GPU-Accelerated Rendering: Ensures high frame rates and smooth animations for large datasets, enhancing user interaction and data exploration.
Integration with Web Applications: Can be embedded in web pages and applications, enabling the creation of interactive and shareable genomic visualizations.
New tools, data, and resources:
GenomeSpy Toolkit: A web app is available at https://genomespy.app and the source code is available at https://github.com/genome-spy/genome-spy under an MIT license. The toolkit is written in JavaScript and TypeScript and supports various genomic data formats.
DECIDER Visualization: Example visualizations and data from the DECIDER clinical trial are available at https://csbi.ltdk.helsinki.fi/p/genomespy-paper-2024/.
Data availability: All sequencing data are available at the European Genome-phenome Archive (EGA) under accession number EGAS00001006775.
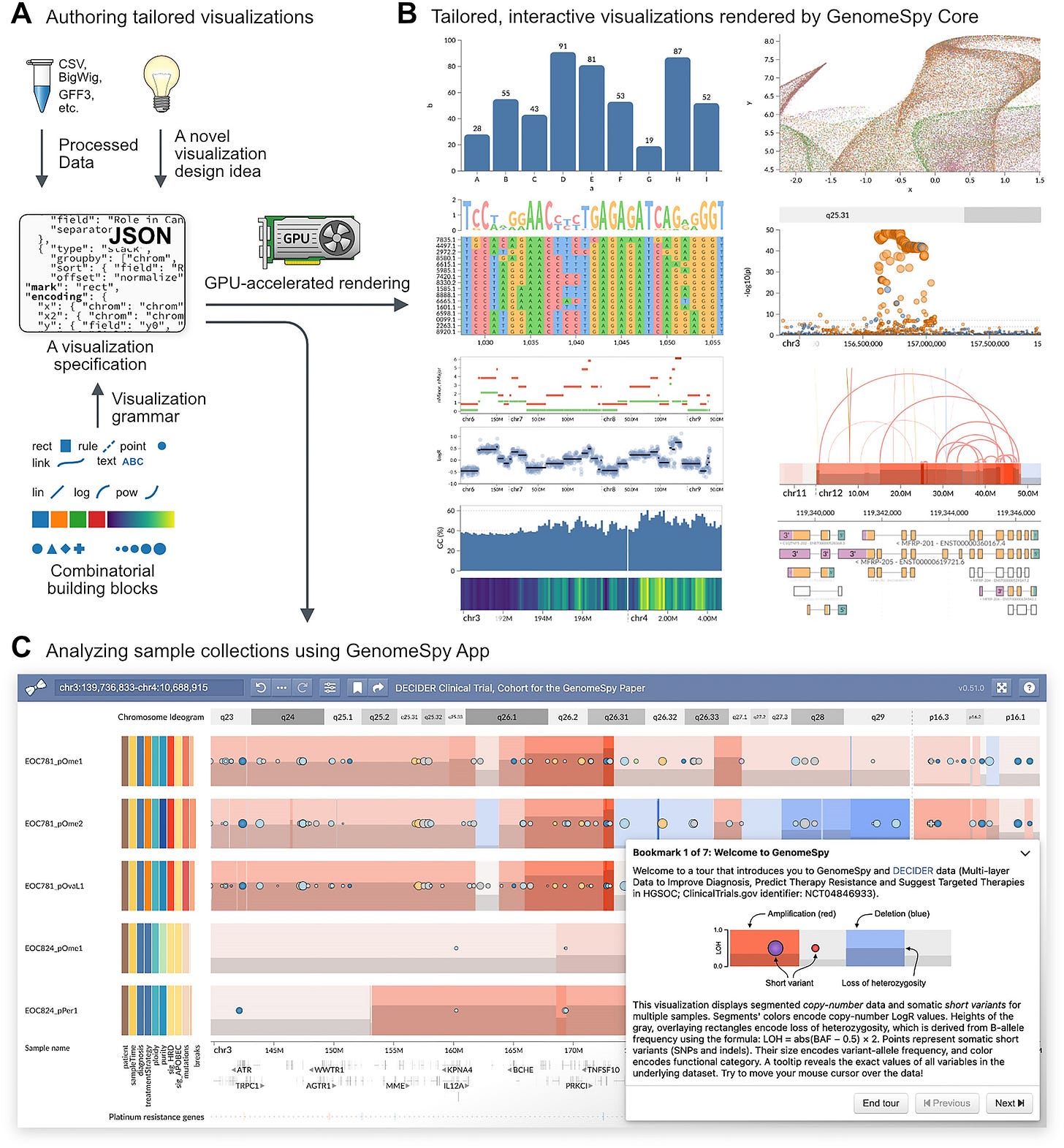
Other papers of note
Current genomic deep learning models display decreased performance in cell type-specific accessible regions https://genomebiology.biomedcentral.com/articles/10.1186/s13059-024-03335-2
Where Are Large Language Models for Code Generation on GitHub? - https://arxiv.org/abs/2406.19544#
SeuratExtend: Streamlining Single-Cell RNA-Seq Analysis Through an Integrated and Intuitive Framework https://www.biorxiv.org/content/10.1101/2024.08.01.606144v1?rss=1 code: https://github.com/huayc09/SeuratExtend
Reconstructing generation intervals over time https://www.nature.com/articles/s41576-024-00766-2 code: https://bitbucket.org/plibradosanz/generationtime/src/master/
A familial, telomere-to-telomere reference for human de novo mutation and recombination from a four-generation pedigree https://www.biorxiv.org/content/10.1101/2024.08.05.606142v1
Early evolution of small body size in Homo floresiensis https://www.nature.com/articles/s41467-024-50649-7
Fast and scalable ensemble learning method for versatile polygenic risk prediction https://www.pnas.org/doi/abs/10.1073/pnas.2403210121
Review: Transformers in single-cell omics: a review and new perspectives https://www.nature.com/articles/s41592-024-02353-z (free to read: https://rdcu.be/dQDdf)
SAFARI: Pangenome Alignment of Ancient DNA Using Purine/Pyrimidine Encodings https://www.biorxiv.org/content/10.1101/2024.08.12.607489v1 https://github.com/grenaud/SAFARI
CaMutQC: An R Package for Integrative Quality Control of Cancer Somatic Mutations https://www.biorxiv.org/content/10.1101/2024.08.12.606123v1?rss=1 https://github.com/likelet/CaMutQC